Is AI the new magic bullet in drug research? Making progress is complex
Artificial intelligence is heralded as the next big leap for drug development, offering the potential to reduce costs, speed up discovery and deliver targeted, more effective treatments.
The hype surrounding machine intelligence has fueled massive investments, with billions of dollars pouring into AI-powered biotech startups.
In China alone, more than 100 AI-driven pharmaceutical companies have emerged since 2018, signaling the country's rapidly expanding role in this innovative space.
Companies like XtalPi, Deep Intelligent Pharma and StoneWise are among the most promising contenders leading the AI revolution in drug discovery.
Despite the buzz, the reality of applying AI to the complex world of drug development is not as straightforward as some would hope.
While AI has made strides in the early stages of drug discovery, its role in later, more intricate phases – such as clinical trials – remains fraught with challenges.
Can AI become the silver bullet for drug development? Perhaps. But the journey to revolutionize the industry will likely take years, if not decades.
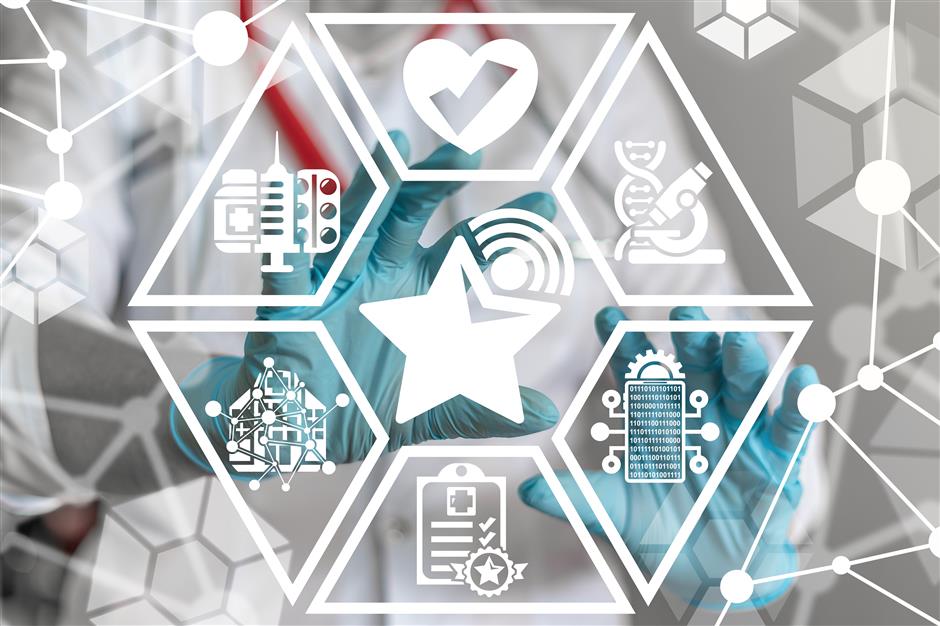
The allure: speed, precision and cost-cutting
The promise of AI lies primarily in its ability to process massive datasets at remarkable speed.
Drug discovery traditionally relies on researchers sifting through vast amounts of data to identify promising molecular structures, predict drug interactions and design optimal treatment strategies.
AI can accelerate these processes by analyzing data faster and more accurately than human researchers, dramatically reducing the time it takes to develop a drug.
US-based Insilico Medicine and UK-based Exscientia, which was bought out by US-based Recursion Pharmaceuticals last year, have already made substantial progress in AI-assisted biotechnology.
Insilico's AI-designed drug for idiopathic pulmonary fibrosis advanced to human trials in less than three years – an achievement that would have been considered impossible a decade ago.
This represents a significant shift in how we think about drug development timelines. AI can turn what was once a lengthy, resource-draining process into something much more rapid and cost-efficient.
AI's ability to cut costs also presents a transformative opportunity for the pharmaceutical industry. The drug development process is notorious for its high costs, with billions of dollars invested before a single candidate reaches clinical trials. AI can streamline this process by identifying viable candidates faster and more accurately, ultimately reducing the financial burden on drug developers and health-care systems alike.
In China, the AI-driven pharmaceutical ecosystem has exploded, with startups and established companies rushing to leverage machine learning to enhance their research and development processes.
Although Chinese firms are still playing catch-up to global leaders in terms of technological maturity, their rapid pace of growth and progress could close the gap in ways that are difficult to predict.
AI hype: a reality check
Despite its potential, AI faces several critical challenges that must be addressed before it can fully revolutionize drug development.
One of the most significant challenges is regulatory approval. The pharmaceutical industry is among the most heavily regulated sectors globally, and for good reason. Ensuring the safety and efficacy of new drugs requires rigorous testing. This process typically takes years, and AI-driven drug development is no exception.
AI has shown promise in streamlining the clinical trial process by predicting success rates, optimizing trial designs and even helping to recruit test patients. AI can sift through large datasets to identify ideal candidates and predict which patients are most likely to benefit from a particular treatment. While this is undoubtedly valuable, it introduces its own set of challenges.
One major issue is the quality and completeness of data.
AI is only as effective as the data it is trained on. In drug development, high-quality, comprehensive data can be scarce. Missing, biased or unrepresentative data can skew results, which is particularly problematic when conducting trials in diverse populations, such as China, where health-care data is not always as robust as in Western countries.
Inaccurate or incomplete data can undermine the results of clinical trials, delaying the approval process or, worse, leading to the approval of ineffective or unsafe treatments.
Additionally, the lack of transparency in AI decision-making remains a major challenge. AI models – particularly those based on deep learning – are often described as "black boxes," meaning that even their creators cannot always explain why the system makes certain decisions.
In high-stakes industries like pharmaceuticals, where patient safety is on the line, this lack of interpretability can be a significant barrier to trust. If an AI system chooses one drug candidate over another, it is crucial for researchers and regulators to understand why.
There are also significant concerns about privacy and data security.
As AI becomes more integral to the analysis of sensitive health information, there is an increasing need for safeguards to protect patient data. Ethical concerns, such as patient consent and algorithmic bias, are also top of mind.
Who owns the data used to train AI models? How are patients' rights and privacy protected? These are questions that regulators will have to grapple with as AI becomes more embedded in drug development.
The future of AI in drug development
AI has already transformed aspects of drug discovery, but it is unlikely to replace traditional methods anytime soon. Instead, the real promise of AI lies in its ability to augment human expertise. AI can accelerate some stages of the process, but it cannot solve the fundamental challenges of drug discovery on its own.
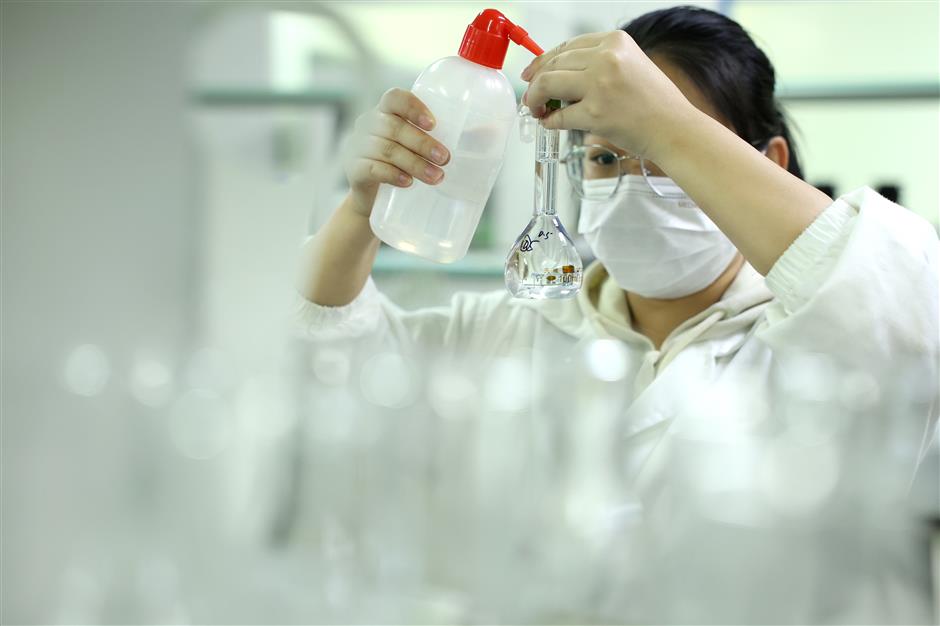
AI working alongside human researchers is the most likely future.
Looking ahead, the future of drug development will be defined by collaboration – AI working alongside human researchers, each complementing the other's strengths.
While AI will continue to drive innovation in drug discovery, human intuition, experience and creativity will remain indispensable. The true breakthrough will come when AI and human researchers work together, harnessing the power of both to unlock new treatments.
In China, as in the rest of the world, AI's role in pharmaceutical innovation will continue to evolve. But it's clear that the road to a fully AI-driven revolution in drug development is long and filled with challenges. Overcoming these hurdles will require time, resources and most importantly, collaboration between AI and human expertise.
(The author is an adjunct research fellow at the Research Center for Global Public Opinion of China, Shanghai International Studies University, and founding partner of 3am Consulting, a consultancy specializing in global communications.)
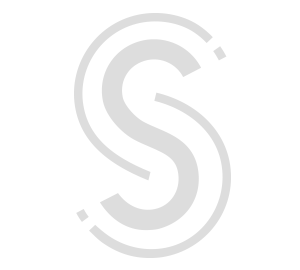