On the importance of professional judgment in the AI era
In collaboration with DeepSeek, a Chinese artificial intelligence startup, Futian District in Shenzhen, south China's Guangdong Province, has in place 70 AI facilities capable of functioning in 240 scenarios ranging from clerical work to emergency management.
Some have hailed these new breed of workers as "chosen," for the employer need not pay them, nor contribute towards their social security accounts, and these machines would not slack off or cut corners on their jobs.
There is also a tinge of unease, however, particularly among the army of undergraduates and graduates swotting up for the civil service examinations: Would civil servant jobs remain so sought after?
The characterization of digital services as "AI civil servants" is misleading, according to local authorities, for while they could help in providing public management and services, they are incapable of independent decision-making. They are essentially just 70 AI accounts that can handle some standardized and basic services.
This deficiency on the part of AI only accentuates the need for human guardianship and vigilantism, throwing into relief the human gift of clairvoyance and intuition, and their capacity for empathy, sympathy, and reflections, which would be forever beyond the pale of silicon-based "life."
As authors Steven M Miller and Thomas H Davenport point out in their book "Working with AI: Real Stories of Human-Machine Collaboration," the majority of sponsors interviewed believed in a model of AI adoption that increased the productivity of workers, rather than totally replacing them.
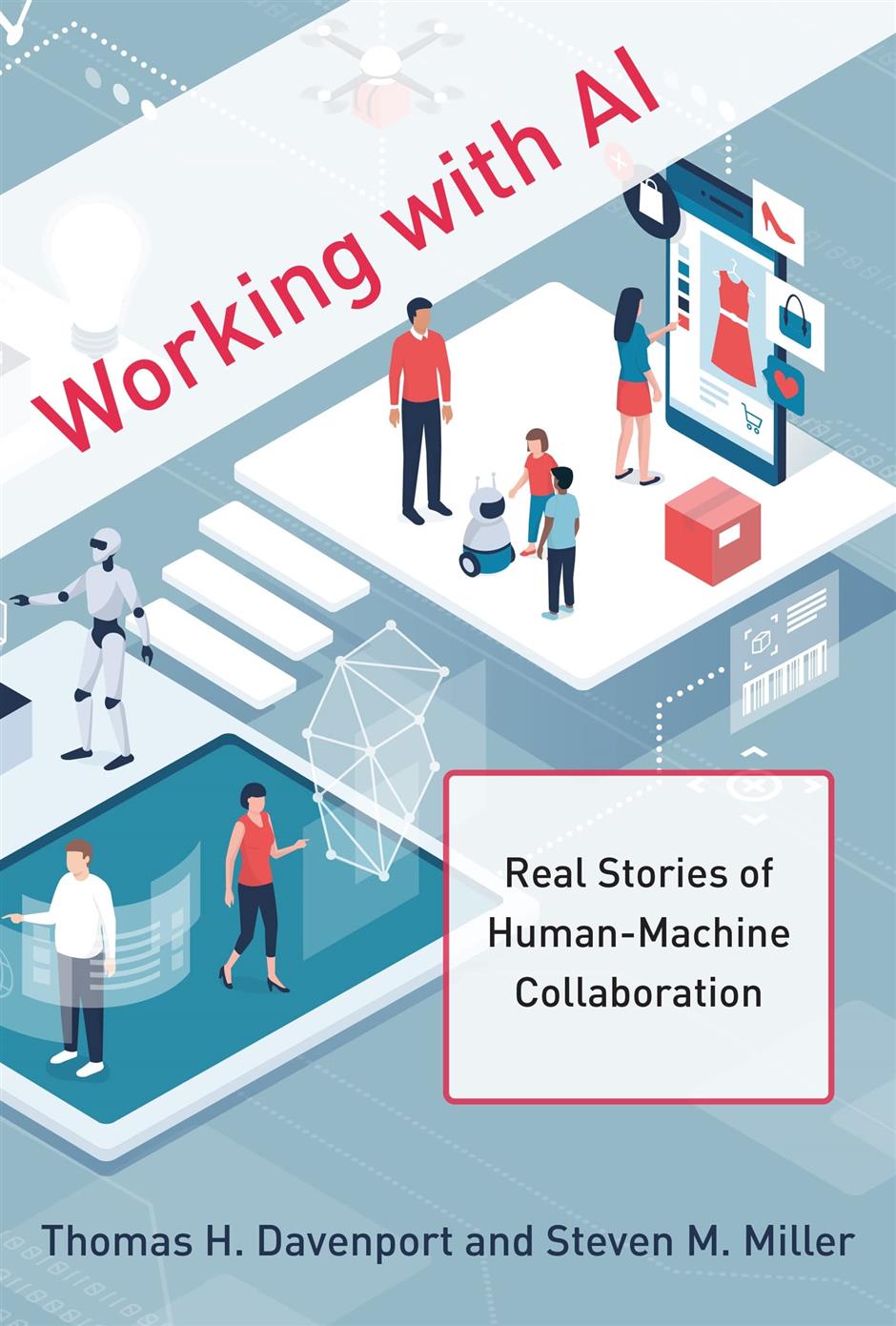
Authors Steven M Miller and Thomas H Davenport's book "Working with AI: Real Stories of Human-Machine Collaboration."
Then, there are still many things machines can't do.
While AI would have some impact on demand for entry-level workers, frontline workers' sophisticated knowledge of their roles is a precondition for successful AI integration.
In the case of the afore-mentioned machines that can handle administrative work for local residents, it is important to be reminded that these AI automation services involve rules-based systems as well as machine-learning systems, meaning the machines are trained on labeled data. The training enables the machines to detect patterns in this data that they then deploy to interpret unlabeled data.
Specifically, the trained systems would be good at such functions as predictions, recommendations, rankings, identifying specific information in a document, but they would be inadequate for other undefined roles, and human workers will be needed to fix the glitches, or other improprieties.
In spite of the apparently inherent risks, the trend for increasing AI automation and augmentation in the workplace would continue.
At Morgan Stanley, a US investment bank, AI is providing personalized recommendations for products and programs based on a client's specific profile and portfolio. This allows for more efficient communication with clients, since AI can effectively tailor recommendations to each client to a degree that human advisors would not have time to do.
But this does not preclude the hiring of human advisors. On the contrary, with the help of the AI system, human advisors are able to give more personalized advice to a higher volume of clients.
At Jewel mall in Singapore, an AI system now partially automates the process of filing incident reports for the security team. The AI pre-fills reports with relevant data and makes it easy to include inputs such as photos and the CCTV footage.
Even though full replacement is not likely, there would be two observable effects on employment: replacement of human work through full automation, or augmentation of human work through partial automation, with the latter dominating.
In a recent Deloitte survey of United States executives, 63 percent of those familiar with AI said they would replace workers with machines to save costs, though their chief motivation would be the help in decision-making by freeing workers, so that they could focus on creative and core tasks.
This might be more of an advantage in aging economies where firms could use automation to make up for a lack of labor supply.
While the overall number of workers needed by firms using augmentation maybe lower, the big picture could be more nuanced, since effective implementation of AI systems relies not just on new technology but also new business models, which might create new roles involved in planning, designing, deploying, monitoring and improving AI systems.
In other words, AI is pushing deeper integration between the business and IT processes, creating a demand for hybrid roles.
Historically, people in business cover activities like human resources, marketing, finance and management, while IT roles cover the creation or configuration of the IT systems people use, as well as data-driven roles like data scientists, analytics specialists, AI/machine-learning engineers and data engineers.
There is now a push to close the gap.
The professional knowledge of frontline workers is essential in the integration of AI into work, where they would be often called upon to evaluate AI suggestions, big-picture thinking, or coordination with other people.
Their professional judgment is becoming more important than ever and, hopefully, more intellectually stimulating.
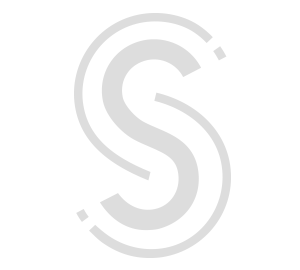